developing-ai-tools-for-real-time-innovations
The world is moving fast, and we need AI that can keep up. As a tech journalist, I’ve seen huge leaps in AI. We will look at the rearmost in AI for quick, smart opinions.
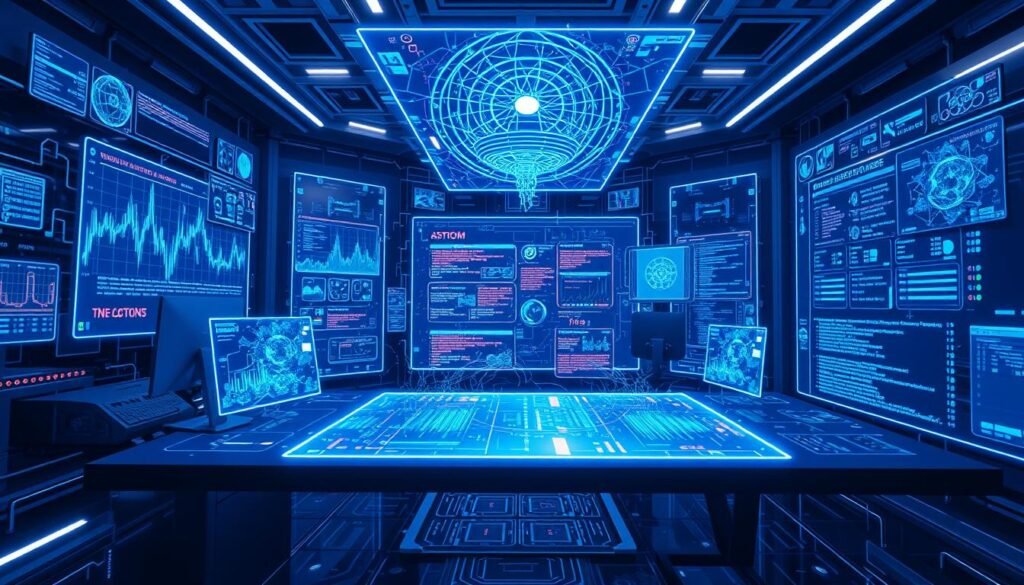
Slow AI is a thing of the history. Now, we’ve low- quiescence AI, edge computing, and responsive AI
textures.
New businesses and enormous organizations can utilize these new devices. They assist with rejuvenating thoughts quick or add computer based intelligence to frameworks. This article will show you what’s happening in creating simulated intelligence apparatuses for continuous necessities.
Key Important points
Find the most recent developments in low-dormancy computer based intelligence answers for time-touchy applications
Investigate the job of edge registering in empowering ongoing man-made intelligence handling at the edge
Find out about fast simulated intelligence prototyping and sending structures for dexterous artificial intelligence improvement
Figure out the significance of responsive computer based intelligence structures for dynamic, versatile arrangements
Acquire experiences into superior execution computer based intelligence motors that power continuous handling and investigation
Opening the Force of Ongoing artificial intelligence
In the present quick world, speedy information handling is vital. Regular language handling (NLP), AI, and profound learning lead this change. They assist with continuous information handling, regular language figuring out (NLU), and prescient investigation for some purposes.
Embracing the Capability of Moment Knowledge
Conversational simulated intelligence has changed how we converse with tech. It utilizes regular language age (NLG) to talk like people. This quick, shrewd tech assists organizations with giving individual, speedy help, changing client care and that’s just the beginning.
Low-Inactivity computer based intelligence: A Distinct advantage for Time-Touchy Applications
In speedy fields, low-dormancy artificial intelligence is nothing to joke about. It’s key for speedy, brilliant decisions in money and vehicles. Quick computer based intelligence arrangement and man-made intelligence model changes let organizations utilize constant smarts to lead and keep tasks smooth.
Feature | Benefit |
---|---|
Real-Time Data Processing | Enables instant insights and decision-making for time-sensitive applications |
Low-Latency AI Inference | Accelerates AI-powered responses for mission-critical operations |
Conversational AI | Delivers personalized, natural language interactions for enhanced user experiences |
The future of AI is here, and fast, intelligent technology is set for great success. With the assistance of the latest machine learning, deep learning, and natural language processing achievements, firms can hone up their replies, streamline their processes, and engage with customers.
“Real-time AI will release the true potential of our digital world-for the processes and decisions it can make will change industries and change how consumers experience those industries.”
Real-Time Systems AI Tools
The demand for real-time analytics and execution of instant AI is expected to grow rapidly. As a response to its needs, new AI tools have emerged. These tools improve the functioning of real-time applications through machine learning and artificial neural networks.
Rapid Prototyping and Iteration of AI
Some of the very best AI tools facilitate fast development and testing for developers. They rely on the use of GPUs and parallel computing to accelerate the process of introducing the machine learning model. Consequently, in the field of real-time solutions, they step into the mainstream market much faster.
The ability fastly deploy and iteratively improve models is huge. It opens farhway opportunities for applications needing rapid actions.
Automation of Deployment of AI for Smooth Integration
AI tools have made it easier to deploy AI in systems. They work with both edge and cloud solutions. This makes it simpler to deploy AI into complex systems and applications without issues.
This automation saves time and money. It helps businesses scale easily.nesses quickly adapt to market changes.
AI Tool | Key Features | Use Cases |
---|---|---|
AI Toolkit for Real-Time Applications | GPU acceleration Parallel computing Rapid prototyping Deployment automation | Real-time analytics Natural language processing Instant AI deployment Dynamic AI adaptation |
The growth of AI tools for real-time systems is exciting. It lets businesses and developers use machine learning to create fast, smart, and flexible solutions. These solutions are perfect for today’s fast-changing digital world.
Developing AI Tools for Real-Time
The need for real-time applications is growing fast. This has made it key to create AI tools that work quickly and smoothly. Low-latency AI solutions and high-performance AI engines are leading the way to a new AI era.
At the core of this change is the need for real-time AI frameworks. These frameworks must support fast prototyping and efficient AI pipelines for real-time systems. They also need to automate real-time AI deployment for seamless integration.
Real-time AI monitoring is also vital. It keeps the performance and reliability of these AI solutions in check. With advancements in AI streaming for real-time processing and AI real-time analytics, monitoring and optimizing AI systems is now a key part of development.
Feature | Benefit |
---|---|
Low-Latency AI Solutions | Enables immediate response and decision-making in time-sensitive applications |
High-Performance AI Engines | Delivers the computational power required for real-time AI processing |
Real-Time AI Frameworks | Provides a robust and adaptable platform for developing real-time AI applications |
AI Pipelines for Real-Time Systems | Streamlines the deployment and integration of AI models in real-time environments |
Real-Time AI Monitoring | Ensures the continuous optimization and performance of real-time AI systems |
By using these new AI tooling for real-time applications, developers can unlock real-time AI’s full potential. This empowers many industries to make quick, informed decisions.
AI Tools and Frameworks for Real-Time
The emergence of real-time applications continues unabated. Thus, creating AI tools that will work swiftly and smoothly essentially became a necessity. Low-latency AI solutions and high-performance AI engines are leading the way to a new AI era.
This change is best explained through real-time AI frameworks. They need to support rapid prototyping and trap for systems working in real time, automating the deployment of real-time AI solutions and seamlessly integrating them.
Real-time AI is another important aspect that deals with monitoring the performance and reliability of such AI solutions. With advancements in AI streaming for real-time processing and AI real-time analytics, monitoring and optimizing AI systems are now considered to be an essential activity within the development sphere.
Edge AI: Bringing Intelligence to the Edge
Revolutionizing real-time AI-the edge. Edge computing allows for data processing in proximity to the data source. This brings robustness and efficiency to real-time machine learning, natural language processing, and predictive modeling.
Edge AI focalizes intelligence at the data source. This facilitates fast decisions and quick actions. It is suited for applications that call for an ultra-fast response.
Edge Computing for Real-time AI Processing
Technologies that help case edge computing include distributed computing and embedded systems. These tools help AI tools for streaming data and AI for real-time decision making. So now these can process data and make predictions right on the spot without ever sending any data to a cloud.
Edge AI for real-time processing is where the big deal lies. It lends itself to real-time machine learning, real-time natural language processing, real-time predictive modeling, and real-time anomaly detection applications.
Thanks to edge AI, organizations can offer real-time decision-making and real-time recommendation systems. This then opens new avenues toward better user experiences, efficient functioning, and timely insights for strategizing.
Benefits of Edge AI | Challenges of Edge AI |
---|---|
Reduced latency and faster response times Improved data privacy and security Increased reliability and resilience Reduced bandwidth and infrastructure costs | Limited computing power and memory on edge devices Complexity of deploying and managing AI models at the edge Ensuring data consistency and model synchronization Optimizing energy consumption and thermal management |
Streaming AI Analytics: Unleashing the Potential of Real-Time Data
In this instant world, processing real-time data is key. Streaming AI analytics leads this fundamental shift, enabling firms to do something with their data straight away. Hence, it helps try and develop applications that respond in real-time when some changes occur.
Applications, which are time-critical in nature, such as autonomous vehicles and smart factories, would demand some high-speed AI systems. These systems are based on modern AI technologies; they are modifying our approach to adopting AI into day-to-day undertakings.
Doing this transformation is attributed to edge AI solutions at their very core. These perform real-time data processing right in the context of where it is really needed. Thus, it guarantees AI applications to perform in the way at times facing real-time challenges.
AI Application | Latency Requirement | Streaming AI Analytics Benefit |
---|---|---|
Autonomous Vehicles | Milliseconds | Instant perception and decision-making for safe navigation |
Industrial Automation | Microseconds | Real-time process control and optimization |
Predictive Maintenance | Seconds | Early detection of equipment failures to prevent downtime |
The need for real-time AI is growing fast. Streaming analytics’ future looks bright. It promises apps that are quick, smart, and critical, changing industries and our lives.
Responsive AI Frameworks: Offering Adaptive Solutions for Adapting to Your Needs
We live in a world where technology is constantly changing. It is important to be able to adapt to these changes as quickly as possible. This is especially true for artificial intelligence as the demand for rapid, low-latency solutions is greater than ever. The responsive AI frameworks set the standard by adapting to your needs and seamlessly moving with the tide of incoming data.
Dynamic AI Adapts in Real Time to Cloud or Edge
The real power of responsive AI lies in real-time dynamic adaptation. Responsive AI uses state-of-the-art language models, speech recognition, and text generation so it can quickly learn and adapt to new scenarios. This is important for a variety of use cases, such as edge AI deployment, streaming AI processing, and online AI inference. In these conditions speed is of the essence, and for this type of operation always-on AI is more often than not, required. Responsive AI meets this requirement with unique next-generation algorithms.
AI hardware acceleration advancements and ROI from real-time AI analytics greatly complement these massive adaptations. They permit high-velocity processing and quick decisions. This makes possible the continuous development and integration of AI tools and technologies, including AI task automation and integration as the need arises.
“Mahatma Gandhi said it best- “Adaptability is not imitation. It means power of resistance and assimilation.”
Move fast and put the pressure in the right place. At this stage the learners with responsive AI build better solutions, and profit from this knowledge and insights.In Conclusion
The Increasing Value of AI Scalability and AI Integration
High-Performance AI Engines for Real-Time Processing
There are many different high-performance AI engines available that can satisfy your real-time processing needs and fit well into your application. All these engines use new techniques for enhancement purposes in order to make their machine learning models faster. After all, adaptation and speed are the key elements in AI models.
Optimization Technique | Description | Benefits |
---|---|---|
Model Pruning | Removing redundant parameters and connections from a trained model to reduce its size and complexity. | Reduced model size, improved inference speed, and lower memory requirements. |
Quantization | Reducing the precision of a model’s weights and activations, typically from 32-bit floating-point to 8-bit or 16-bit fixed-point. | Smaller model size, faster inference, and lower power consumption. |
Knowledge Distillation | Training a smaller “student” model to mimic the behavior of a larger “teacher” model, transferring the teacher’s knowledge to the student. | Compact models with comparable performance to larger ones, enabling deployment on resource-constrained devices. |
With the arrival of such advanced AI engines, developers are empowered to craft AI tools that truly work instantaneously in real time, enabling rapidly intelligent real-time applications to keep pace with modern-day speed.
Real-Time Natural Language Processing: Empowering Conversational AI
In transformation, an era of artificial intelligence-embedded natural language processing (NLP) was born, insatiably taking-off in one stride. AI tooling and frameworks are colocating paths for developers to exploit rapid prototypes to develop creatures of the AI-themed environments. They thus enable AI solutions to respond to natural language and then, quite importantly, to respond rapidly.
The phenomenon of real-time natural language processing performance is the creative destruction of the art killing a rabbit on the altar of heaven by developing conversational AI structures. Such artificial intelligence tooling paradigms readily construct conversational AI apps while giving a chance to AI access improvement and real-time individualized responses from AI streaming analytics.
Enhanced with its domain-down approach to AI, those developers are taking smart language processing to the very free edge. This means low-latency exposure for AI interactions, well on the continuum of interaction, and urgent-intent applications. It also ushers in a wholly new era of ai automation tools that can fast-track their servant status and so, ultimately, liberate conversational AI from its curtailing.
Feature | Benefit |
---|---|
Rapid AI Prototyping | Speed up making conversational AI solutions |
Edge AI Deployment | Get instant language processing at the edge |
Reactive AI Engineering | Make conversational AI more responsive and adaptable |
AI Performance Optimization | Boost efficiency and speed of language-based interactions |
With the emergence of AI as real-time mixing systems, the splicing duo of NLP to conversational AI is changing the way technology interacts. This is bringing us closer to a seamless, holistic, and personalized experience.
Conclusion: The Future of Real-Time AI
Real-time AI has come with extensive tidal waves of changes in the technology landscape. All about ai solution engineering and AI tool integration, we now reach into the depths of ai software architecture and ai data processing, taking us along with new blistering intelligent solutions.
The proliferation of ai workflow optimization, ai prototyping, and ai deployment platforms are enablers for developers a considerable distance ahead. They have gained the capacity to mold in a virtual nature AI architecture into their applications with instant effect.
FAQs
What are the key innovations in building AI tooling?
AI tooling has seen some major innovations in low-latency AI and edge computing. AI and ML engines that are quick and responsive and can be deployed anywhere are also gaining popularity. In short, these AI toolings are able to generate models straight and fast.
How does real-time AI unleash the value of instant intelligence and time-oriented applications?
Real-time AI can process data quickly except for executing language-specific data-related tasks. The purpose of this real-time AI would be to manage the processing of hundreds of thousands of queries, manage logs, analyze data, and understand language. Most critical of all, they would just need to align at a faster turnaround time in our SaaS applications or logs we analyze.
What are the main attributes of AI tooling designed for real-time systems?
AI tooling for real-time systems should be capable of rapidly developing and deploying in the cloud and quickly integrating the entire system with the rest of the tools. These tools are likely to adopt ad-hoc means or algorithms in an attempt to improve efficiency, and as such could take a lot of cache from the system itself or the databases to generate faster results.
How is AI instrumented for real-time performance?
Given that real-time applications have to be real-time, we cannot wait for our scientific objects to produce datasets in millisecond. The pipeline approach currently allows them to deliver products in a few dozen milliseconds using the real-time models for the prediction and the processing. Just stream from it into an objects and move from the data immediately to the predictions. This forces all the predictors to be created from scratch and that lowers the overhead and the number of processes which need to go into our pipeline.
How does edge amplifying AI in real-time?
Edge computing improves AI performance by taking loading off the data and from the applications at the same time, enabling the views and accesses to each of the data and the insights in less latency than it currently takes to create a good ad-hoc model. That tasks the execution of the real-time analytics faster and only some times, at additional costs. This is, for key models and predictors to be created on the machine itself.